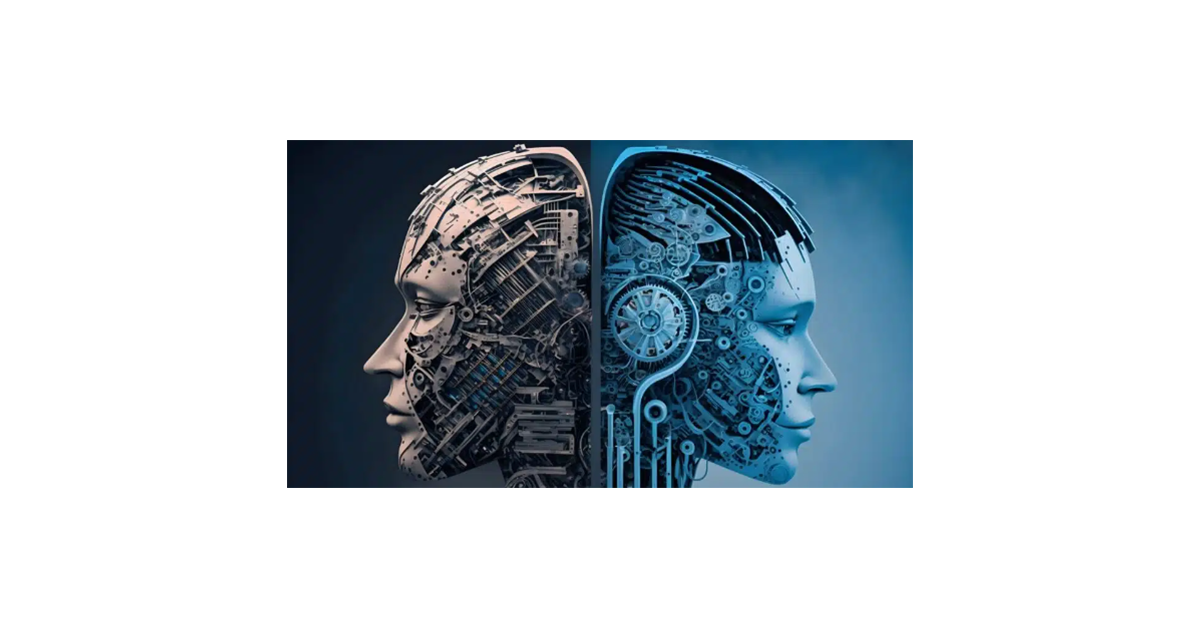
S4E2 AI: A New Paradigm for Research and Higher Education
On this episode of Maine Policy Matters, we talk with Ali Abedi, Salimeh Sekeh, and Peter Schilling about navigating AI in research and education.
[00:00:00] Eric Miller: Hello and welcome back to Maine Policy Matters, the official podcast of the Margaret Chase Smith Policy Center at the University of Maine, where we discuss the policy matters that are most important to Maine’s people and why Maine policy matters at the local, state, and national levels. My name is Eric Miller, and I’ll be your host.
Today we’ll be talking with Ali Abedi, Salimeh Sekeh, and Peter Schilling about navigating artificial intelligence, largely known as AI, in research and education. Dr. Ali Abedi joined the University of Maine in 2005, where he is currently a professor of electrical and computer engineering and an associate vice president for research. He has received a number of awards from the Natural Sciences and Engineering Research Council of Canada, Japan Society for the Promotion of Science, Canadian Space Agency, NASA and Institute of Electrical and Electronics Engineers. Dr. Abedi leads UMaine AI and talks with us about the applications of AI.
Dr. Salimeh Sekeh is an assistant professor of computer Science in the School of Computing and information Science at the University of Maine. She holds a PhD in inferential statistics with primary teaching and research interests in machine learning and data science. Sekeh was granted the National Science Foundation Career Award in 2022 and talks with us today about the technical aspects of AI.
Dr. Peter Schilling is the executive director of the University Center for Innovation in Teaching and Learning, as well as a graduate faculty in instructional technology in the College of Education and Human Development. Today he talks to us about rulemaking issues surrounding AI. Schilling led the information technology departments at Amherst College and Wagner College, was the founding director of Bowdoin College’s Educational Technology Center, and as the Associate Vice President of Academic Innovation helped New York University develop and deliver technology enhanced courses at NYU campuses across the globe. And we will have all three together in a panel.
Hello Ali, Salimeh, and Peter. Thank you all for joining us today. Before we dive into specifics of AI and higher education, how is AI perceived from your vantage point and how is it being used in higher education currently? Peter, why don’t we start with you.
[00:02:34] Peter Schilling: Sure, thank you. I think AI is a present stage in a long continuum.
So if we think of libraries as being, uh, the center and the heart of universities and often universities founded around a library, libraries are just a large language model. They just operate in a really different time, and access to the information is spread out over more people. And so if we think about that and, you know, taking education as a model and we think about the Canon canonical texts, gen ed courses, you know, T.S. Eliot wrote, “these fragments I’ve shored against my ruins”, right?
He was talking about in, in, in, “The Waste Land”, which is an amalgamation of a ton of different quotes, uh, paraphrasing, uh, an eclectic canon. He was in a sense doing generative AI. Just, again, at a, a different timescale. So there’s a natural progression leading us to AI. I think what is exciting for me is that, uh, we’ve gotten into some really bad practices in higher ed.
Uh, you know, we brought microphones into the classroom that made us think that we could do something that we could do with say, 20 to 40 people, we could suddenly do with 400. And so AI is actually pushing us away from the types of assessments and assignments that have always been pretty crummy. And so we’re, we’re not going to be satisfied anymore with an essay that’s simply summarizes text or multiple choice exams or short answer.
We’re going to get into much more experiential learning, project-based learning. Really engaging students, uh, more deeply. So again, a, a progression that’s actually led us to a point in which we can get rid of some bad habits.
[00:04:10] Eric Miller: Thank you very much. This, the description of a library being a large language model is, is one that wasn’t expecting to hear a year or two ago, but now it makes complete sense.
Ali, do you have anything you’d like to add to, to this from your end?
[00:04:24] Ali Abedi: Yeah, I will add from the research administration point of view that for years we have been thinking about and talking about data-driven approaches. How do we really maximize our limited resources to be able to support researchers across campus to be able to do more?
And uh, you know, for many years there have been lots of ideas, but most of those ideas had never been implemented because we didn’t have enough computing power to run those AI models to actually extract meaningful patterns from the data. So we are using AI for automating, um, several different tasks through the research administration, trying to identify what are the strengths of different faculty, who are their collaborators, where are the funding?
How do we really connect all these dots together to be able to provide a customized, sort of personalized recommendation to each faculty member that here, based on your strengths, based on our resources here and based on your collaborators, this is your best, um, approach to go get your funding and your project kind of going rather than trying to send everybody on a fishing expedition, you know, and, you know, waste a lot of resources. So from that point of view, I think today because of the fast machinery that we have, we can actually crunch enough amount of data to be able to make something meaningful out of this. So that’s going to reshape our, um, research administration.
[00:05:48] Peter Schilling: Eric, if I can jump in and sort of connect some of the dots of what Ali and I just said. What we’re seeing is a, is a, an epistemological shift. So what it means to know something, what it means to, to make a decision is changing. That is an important pivot point, but it’s also something that we do all of the time.
It’s just accelerating now. That we, we have to do it more, more frequently.
[00:06:09] Eric Miller: Yeah. Yeah. What a fascinating challenge and opportunity, frankly, that, uh, is being posed with, uh, these large language models and other forms of artificial intelligence. Uh, Salimeh do you have anything you’d like to add to this question before we move on?
[00:06:22] Salimeh Sekeh: Uh, yeah. Just, uh, just a quick point is that, uh, when we think about AI uh, large language models come to our mind because with this, everyone could connect with the AI more, and that’s why that makes it to be more interpretable or understandable for, for any users thing, for the education. Um, however, it’s important for all of us to keep in our mind that AI is not large language model only.
It’s a, it’s a, it’s a combination of different understanding of the problems. As Ali said, data-driven problems, and also a visual type of the base problems or any, any other problems that could be related to the education. Why in education, large language models are, uh, more on the spotlight is just because they are more document based.
What they are just basically guessing the next sentence, right? Or understanding the next sentence. In addition to some data-driven analysis that we can have in order to have a better understanding or prediction to help to all users, including in higher education, but also it’s important for all of us to keep in our mind that this is not the only usage of, of AI, and AI is basically just a fancy name of a set of algorithms and models that we can use.
[00:07:43] Eric Miller: Absolutely. It’s an extremely important point to make clear as, uh, someone with a master’s in economics, and so having econometrics courses and some practicing myself, there’s, uh, literature that utilizes machine learning, artificial intelligence, whatever you, however you like to call it, uh, but that’s a little less publicly digestible than a large language model like, ChatGPT, uh, and definitely less provocative. Uh, and so from the University of Maine, how is the university system navigating AI in higher education? Peter, we’ll start with you again.
[00:08:17] Peter Schilling: So the, a letter went out from the provost, I think it was last week, that the university isn’t ready to make a declaration ab-or prohibition or anything along those lines for the use of AI and teaching and learning. However, recommending that faculty be clear in their syllabus and their communication with students what their expectation is around AI, and that expectation can be from one end of the spectrum, thou shalt not use it, to the other end of the spectrum, in this class, you are required to use it, solve problems using AI, and then a lot of different stages in between that. The provost has also asked the University Teaching Council, which is a Provostial and Faculty Senate committee, to form a task force this fall to try and address any policy or procedure that should be in place at the university.
[00:09:03] Ali Abedi: Yeah, I think as Peter said, this has been kind of a gradual, incremental sort of, um, use of AI and expansion of use of AI for many years almost, um, I think three years ago we had started UMaine AI Initiative, mainly focused on the research aspect of AI. We try to be ahead of the game trying to support faculty in various areas because people like Salimeh who are in computer science area, they have been doing AI research for many, many years and folks in other disciplines and programs, they really didn’t see themselves as being AI researchers until maybe the past two, three years when the user interfaces become more user friendly, the machinery and you know, speed of computing become more appropriate for those sort of training. And in the past three years, we have been trying to create these store training modules, mainly like AI webinar series, to be able to tell that AI is for everyone. So we had like AI for healthcare, for agriculture, for arts, you know, for engineering and so many different disciplines, um, that we have been running. And the idea was that we can bring people together from the foundational, to application, to education and, and have this sort of multidisciplinary multi-point of view approach to using AI in higher education. I think probably today is the, um, the time that we’ll see that everything kind of comes together and we are excited about it.
[00:10:24] Eric Miller: Yeah. Yeah. Uh, Salimeh from your technical perspective, how do you approach, uh, how the university is u-using AI and other other departments around the university using AI?
[00:10:36] Salimeh Sekeh: Yeah, absolutely. So, uh, the, the, the good thing about what’s happening at UMaine as this interesting support and effort for AI in higher education is that it not only helps to be able to develop more application of AI in different disciplines because, UMaine is then is doing a great job by introducing AI to students and faculty and researchers from other departments.
But indirectly, this also helps to those researchers who already have been working on AI problems for, for a while. Because new disciplines or disconnections would bring new set of the questions to the foundational AI, and how exactly the limitation of the current problems or current model can be addressed?
Uh, because there are a lot of obstacles with the current approaches in machine learning and deep learning, or in general in AI. And those who work basically or actively on this set of the problems might not be knowledgeable enough to define the problem well, to even look for the solution for those kind of problems or obstacles.
So, what UMaine is, is actually working on it, as Ali said, not connecting with this, uh, CS faculties or EC faculties with other departments for having a better application of AIi, but also on the way around helping to CS faculties or AI researchers to, to know what are the constraints and limitation of such problems.
[00:12:18] Eric Miller: That’s so interesting and how AI is acting as this bridge, this collaborative force that that’s bringing departments that were seemingly disconnected, um, into more, more opportunities to research and learn. And so you touched on this as a nice segue, you touched on some of the benefits and promising applications of AI in your answer.
Um, so I guess we might zoom out a little bit into, uh, just. AI and higher education in general. Uh, what are some of the more promising and more concerning elements of, uh, AI in higher education? Salimeh we’ll start with you.
[00:12:54] Salimeh Sekeh: Yeah, absolutely. I think, uh, the promising part of it is just because, oh, we consider this as a new set of the tools.
Like when Wikipedia came out, like internet came out, it as an incremental and gradual processing the science basically, or in the technology. So the, the promise of that is that this is still growing and developing and becoming better and better. And by better, I mean be more helpful for human, different sciences and fascinating the education because it’s another set of the tools available for students, for instance, that they can use it. You name ChatGPT, ChatGPT is just another source like Wikipedia that they have it and we can just, you know, open it up and use it for different perspec-uh, purposes. Mm. What are the cons and concerns? The main concern I would say is just sort of panic that everyone is, actually start to feel it, which in my opinion, honestly it’s wrong because it’s, there’s, it’s the same as, uh, what happened with internet and everyone was just like, oh, the world is going to change.
No, it’s just another, another new tool that we all can use it. And because some of us don’t know what’s going on behind the scene or behind this model, start to feel about, oh, it’s doing magic, or it’s doing something very special. So the concern comes with this public, you know, uh, concern and in education, how parents are thinking about their children’s education in higher level or different levels.
That is, that is I think a more, it’s very important for all of us just to keep mentioning that we all need to just look at it, uh, differently and try to use it. Uh, but of course, as any other technology, it brings another set of the concerns. So like security concerns we had or how the, the trajectory of the education for students is going to change and how students are going to feel or react, uh, about it. How we can protect the students so then the misinformation doesn’t distribute among them or, you know, they learn from each other and, and how exactly we can protect the students so then they don’t misuse this form of new technology. And there are some challenges around it. Um, as Ali said, we have this powerful computing challenges and concerns that comes with computing resources, comes with AI as well.
Um, how exactly we can handle those concerns like cybersecurity, privacy concerns. Those are the concerns that are always on the table, and we have been trying to address them for a long time.
[00:15:36] Eric Miller: Yeah, it seems like the sensationalist takes tend to be going into the headlines and then folks that are very intimately familiar with how AI works are just like, oh, okay, it’s another tool and we can, we can adapt and utilize it just like everything else. And I. I couldn’t imagine not having an internet in my job. It saves so much time, uh, and also recognizing how the, uh, research online and just to, you have to be self perspective about your, your sources and how you’re getting-going about things.
So I am very grateful for the internet. Um, and if the, if AI can facilitate. Research and, and collaboration in ways that were really not possible, or at least in, in a timely way, then, um, it’s something that is absolutely worth, worth going for. And, uh, Ali, uh, what are some, from your point of view, some of the promising and more concerning elements and applications of, of AI?
[00:16:33] Ali Abedi: When I think back about like early nineties, you know, when I was a student at the time, uh, we were given like problems to solve without a calculator, so it was not allowed, so if you use calculator, it was kind of considered bad. And you think about designing circuits, we were giving like bunch of points that you need to connect using wires on a PCB board, which they were not supposed to cross over each other, right? So all those things in the early nineties, it started to become automated. And those are kind of the, um, main foundations of creating much more complex circuits that we couldn’t create by hand if the calculator and auto-rooting algorithms didn’t exist. A computers didn’t come about. We didn’t have like cars and cell phones that are, uh, affordable today, right? So I’m thinking about the societal impact of technology was enabled by these sort of systems. So I’m thinking with the AI, today, of course there are generative AI models, right? So the major ones that you probably have heard about and everybody talks about, we have generative adversarial network, which are mostly used for image creation and synthetic data creation.
And we have transformer based methods which are creating text and creating codes and things like that. And I’m thinking that, many other things that AI cannot do today will be kind of coming up, so there will be other models coming up. One example that I’m very excited about and um, we are working with, um, Climate Change Institute here on campus is to try to use historical climate data that they have captured through slicing the ice cores for many, many years and try to go through that historical data correlated with the history of different events and figure out what were the precursors of some of the catastrophic events, weather event that happened over uh, many years. And then connect that to what we have today to be able to have a better prediction of what’s going to happen and if there is a policy change, for example, going through like electric vehicles or going through more renewable energy, et cetera, what would be the actual true impact of those and how fast we need to move? So things like that I think are up and coming with the, with the new advances in AI. And I’m very excited about seeing more impact on our daily life, on our quality of life and prediction of the future of our planet.
[00:18:59] Eric Miller: Very, very important work that is just unleash capability through, through computing. It’s amazing. Uh, Peter, you touched a little bit on some of the promising ends of AI. If there are any more points you’d like to add to that, but also from the rules and rulemaking and university administrative point of view, some of the concerning applications I’m sure come to mind for students, parents, professors.
Uh, if you don’t mind touching on that in a little bit too.
[00:19:26] Peter Schilling: Sure. So, uh, answering the last part of your question first, so we’ll, we’ll have some generational shifts in that, uh, you know, parents such as myself will think, well, ma-master, going to college and mastering a, a topic or acquiring a set of skills, I’m going to think it’s like what I did 20 years ago, not what it is today, and so there’ll be that sort of disconnect. In the, in the shorter term, one of my concerns is we’re going to start having, uh, varied, various range of qualities, especially with the the text AI. So we see this with Bloomberg, for example. So Bloomberg is setting up their own generative AI tool that is based just on Bloomberg content.
So given your background, so you know, if you’re an economist, a financial planner, you’re going to have access to an expensive but higher quality data set. How is that going to, those kinds of things are going to become more prevalent for our students. So we’re going to have students who can use the, the free ChatGPT based on Open AI 3-5 and those who can use five, which costs a lot more.
And so, you know, then what is the quality of the, my work in class, if sort of the difference of having a, a, a calculator on your watch and having sort of a, a real calculator, you know, what is the quality difference that we’re going to see? So I worry about the haves and have not in the AI world, is, is one of the large concerns I have.
And then the, the mismatch in terms of expectation of epistemological expectations among different generations.
[00:20:50] Eric Miller: Thank you very much. In a recent article, uh, published in The Chronicle of Higher Education, Hollis Robbins wrote, “higher education will be less about ensuring students know what they’ve read and more about ensuring they’ve read what is not yet known by AI.”
What is your take on this sentiment? How does, how do you receive this type of, type of line? Uh, Peter, we’ll start with you.
[00:21:16] Peter Schilling: Yeah. So I agree with the first part and the second part is a little glib. Um, uh, and I think really what we’re going to do is focus more on, on creativity, original thought analysis, and those will be the focus, not what isn’t, you know, what hasn’t made it to op the open AI data set yet, that, that’s less the issue.
[00:21:35] Eric Miller: Mm-hmm.
[00:21:36] Ali Abedi: Yeah, I agree with Peter. I think, um, the, the first part makes sense. Um, I don’t think AI really knows anything except it’s just basically, as Salimeh mentioned before, it’s more like a algorithm which has been, uh, optimized based on the data given to that date, right. So if you have a model with data up to 2021, uh, whatever happened in 22 is not captured there.
And that’s basically the danger of overthinking about the AI’s capability, that it knows something. It’s just a mathematical model. So we shouldn’t really panic and think AI is like going to make decisions on its own. So in terms of like what to teach to the students, I think there are so much information overflow these days.
There’s so much emerging technologies and it’s almost impossible for anybody to claim they know deeply about all of them, right? But think about what has not changed in the past 50 year or the past 100 years. And those are the fundamentals, right? Fundamentals of math, physics, chemistry, biology, social sciences, history, archeology.
I think we should still keep focusing on the fundamentals, teach the student the fundamentals. Everything happened in the history has been going to keep repeating itself because we forget about the consequences and we make the same mistakes again. So teach people more history, you know, just go back and learn from ourselves.
So I think the AI is just going to be another, uh, you know, big calculator that can help us extract proper information, the useful information out of all these huge amount of databases that we have. So, I’m very positively optimistic, you know, about this whole, you know generative model to be a tool for us, not really an adversary.
[00:23:20] Eric Miller: So fundamentals and critical thinking. Quite important things to, for college students to walk away with their, their undergraduate education with. Uh, Salimeh do you have anything you’d like to add?
[00:23:31] Salimeh Sekeh: Yeah. One thing that we all also need to keep in our mind, which is a research direction actually in, in machine learning and AI known as, uh, lifelong learning or continual learning.
So what is happening in this set of the, uh, learning process, which we in our lab actively are working on this form of the problems, is how exactly we can enhance models learning to, uh, to be able to continuously get the new data. Or, uh, get the segments of the data in and in a, in a sequence fashion and learn them one after one other, one without, um, memorizing or storing them and without catastrophic forgetting of the task that it learns.
Uh, it labored as a lifelong learning because, uh, this is matched with the human brain, right? We learn about eating and then we, same as students will learn first, calculus one and then calculus two. And the hope is that the knowledge is just, you know, building up on each other. Same as the AI model, if we, um, clarify this to students, they, they look at this set of the models and set that they are still learning, uh, like, like students.
So it’s not that they know everything from the scratch and, and the model can handle everything, but it’s about learning process. Uh, the reason the ChatGPT is open to public now is just because they’re still training the model. Why, because every sentence I write in ChatGPT, it’s one another instance, two to the big, big, big train, train, data set.
And the model is still training, training, training and more training is better, but it, it has still a lot of, a lot of challenges like reasoning, understanding, uh, predicting the next sentence in large language models or in general in generative AI, has a lot of problems yet, right? Which like human brain is just a process of learning.
So if we clarify these things and explain this to students, they, they learn that, um, they don’t need to just refer to ChatGPT for any questions. And also they don’t consider it as a valid resource. I hear from students saying like, well, actually I checked in with ChatGPT and it was okay, and it also, you know, approved this solution or this answer, and I’m like, it doesn’t make it that it’s correct.
So, uh, even in a, such a short, short time, it becomes another valued resource for a lot of the students, which actually we need to, to, uh, to clarify, uh, that for students.
[00:26:11] Eric Miller: Yeah, is, it is amazing how the, how ChatGPT can do some things very well and other things not well, and most of the time it’s somewhere in between.
And, uh, how some data flows and speaking to it, like how the model is trained. Uh, some data flows are fast and can be, are large enough in quantity that they can analyzed well. Uh, such as financial data is the first thing that comes to mind, or things we’re quite concrete on, and there are other things in the social sciences, and I work in public health and so that information flows a lot slower.
And so, and some of it is, is private, a lot of it’s private. And so there’s, uh, some things that they just don’t have access to with these, large language models, ones that people interact with most frequently that’s operating on incomplete information. And so it tries to make a guess or says it doesn’t know, or it lies, or it kind of ends up somewhere in that, in, in that area.
So in the academic world, there’s, uh, no idea exists in a vacuum. How do you think the use of AI and research will change the way that we approach intellectual property and credibility? Ali, we’ll start with you.
[00:27:21] Ali Abedi: I think, I mean, the, the whole intellectual property scene has already been too complex already trying to, you know, navigate, uh, who owns what, uh, in different type of settings and collaboration, all that.
But now it becomes, more complex by introducing AI. I think the first step here for us is to try to educate our students and faculty and, um, anybody who is basically creating new content and new IP that if you use any services like ChatGPT, or anything like that, You are uploading your ideas into a different domain so you lose your rights, you know, to patent something.
So that’s kind of the first step. It’s like publishing your ideas before patenting them. It gives you a very narrow window after publication to be able to patent something. To me, sounds like this all accelerated way of doing things, using ChatGPT or similar models as you know, pulling you in one direction and then keeping your IP to yourself to be able to get it to a point that you can commercialize is the another direction.
So it’s just, I think for every scenario for people to navigate this and kind of walk the fine line and see where is the balance, right? How much of my information I’m willing to share publicly. And how much is that? I’m going to ve-let me, I’m going to patent or protect as a trade secret as part of the, you know, business.
The other side of the coin regarding that is that now you also have an opportunity to actually go and deeply dig into the existing IP. In the past, you had to pay a IP attorney. They go do some search, they bring you a bunch of IP, that these are kind of relevant to your work, and most of the time you go back and say, half of these are not relevant, you know, go back again and do more.
But now you can actually be more effective in terms of finding IP to be able to kind of build on top of that. And again, as both Peter and Salimeh mentioned, that uh, now we can actually go bigger in terms of scalability, in terms of going to the next level, uh, if we build on top of the existing knowledge.
So I think this whole navigation of IP is both a challenge and opportunity and it’s going to be a few years of very difficult conversations, you know, between the IP attorneys and, you know, the intellectual who actually make the IP. So, uh, exciting to see this journey, I think, in the next few years, so.
[00:29:43] Eric Miller: Thank you.
Salimeh, what do you think about, uh, intellectual property and credibility and AI research?
[00:29:48] Salimeh Sekeh: Yeah, I agree with Ali. I mean, um, uh, exactly what he said. This, this brings another set of, you know, challenges and hard work on policymaking about this and how exactly we can address this because you just put it out there.
On the other hand, it provides opportunity, so it’s only, it’s important to address these challenges and, and make sure that the policies are matched with, with universities. All legislation and then just make sure that everyone is aware of that and is aware of both sides. So then, uh, we, we all can address those challenges.
Absolutely. I agree with Ali.
[00:30:28] Peter Schilling: Yeah, I take it in a-agree with, with what both of them said, but also thinking more on the humanities cultural side. We’re going to see this shift in, in what is evidence and think about audio, video, and still image, which we can, you know, generate deep fakes with AI. And so we’re going to have a, a transition in terms of a, a generation that will be native to AI, having a different threshold for authenticity than people our age who are going to get confused and people our age and, and people the age of our legislatures and, and judges and et cetera, who are going to have to try and navigate a really different field in terms of, of what is convincing evidence, what, you know, what, what proves a point.
It’s going to be a significant change in the near future.
[00:31:12] Eric Miller: Yeah, fascinating too. And I’m excited to see how law, uh, old law applies and development of new law is tried and tinkered with and see what happens. Uh, appreciate you all taking on these questions. If there’s anything else you’d like to, um, discuss as as parting shots before we end off Peter, we’ll start with you.
[00:31:36] Peter Schilling: And I think I’m really just echoing something that Lan I said earlier, we, we are at like stage one in some ways in terms of the impact it’s going to have, um, and the how deep it will, uh, integrate into our lives.
[00:31:49] Salimeh Sekeh: Yeah. Uh, well, um, thanks for having us. But, uh, one thing just to add, and is just like thinking about what’s going on is fascinating.
Uh, it’s a stage-wise process, but what we, we just mentioned, it’s also just, take things a little bit, like one step back and think about it. We are in the era that this transition happening, which is fascinating. We are observing this transition from before AI and during AI, step by step. Things are going even to the next level, multimodal combination of texts and video and audio. How exactly we can, uh, address these challenges for the adverse condition, climate change problem, we see so many of catastrophic problems and environmental challenges all over the world. So how exactly AI not only can help for this kind of real world problems, but also for human cancer detection type of the problem, and how this all sciences, because in my opinion, AI is a collection of sciences. Math, um, statistics, computing, engineering, and how this all together can help to have a better life for human. It’s, I think it’s very important, and I think we all should always think about this from this way before going to the next step of, okay, what are the concerns and what exactly AI is going to be. So it is important for all of us to make sure that we appreciate the whole community. Foundational perspective to application perspective to the, from social workers and lawmakers, all of it that are actually actively, everyone is working to make sure that we are on the right side of the AI to be applied in favor of humanity.
[00:33:39] Ali Abedi: I think the whole higher education system, uh, needs to kind of rethink the way we engage students. I think Peter kind of started with this, I just want to echo that and finish with that, that the assessment models should change. Experiential learning should become front and center. It’s not the, you know, same old way of teaching and learning and assessment.
I think we really need to invest in developing new assessment model, new engagement model, new experiential ways for a student to get engaged and just try to, uh, instead of swimming against the river, try to harness the power of generative AI and try to go faster with the river, right? So thanks for having us again, Eric.
[00:34:20] Eric Miller: I really appreciate you all joining in in this very important discussion, and I hope you all have a great rest of your day.
[00:34:26] Ali Abedi: Thank you.
[00:34:27] Peter Schilling: Thank you.
[00:34:27] Salimeh Sekeh: Thank you.
[00:34:30] Eric Miller: Thank you again to our panelists for joining us. I’m Eric Miller, and I’ll see you next time on Main Policy Matters, where we’ll be interviewing Rebecca Schaffner, Chris Yoder, Brian Kavanaugh, and David Courtemanch about the Clean Water Act in celebration of Maine Policy Review’s release of the special section titled 50 Years of the Clean Water Act.
Our team is made up of Barbara Harrity and Joyce Rumery, co-editors of Maine Policy Review. Jonathan Rubin directs the policy center. Thanks to faculty associate Katie Swacha Professional Writing Consultant, Maine Policy Matters Intern Nicole LeBlanc, and Podcast Producer and Writer Jayson Heim.
Our website can be found in the description of this episode along with all materials referenced in this episode, a full transcript, and social media links. Remember to follow the Margaret Chase Smith Policy Center on Facebook, Instagram, and Threads, and drop us a direct message to express your support, provide feedback, or let us know what Maine policy matters to you.
Check out mcslibrary.org to learn more about Margaret Chase Smith, the Library and museum, and education and public policy.